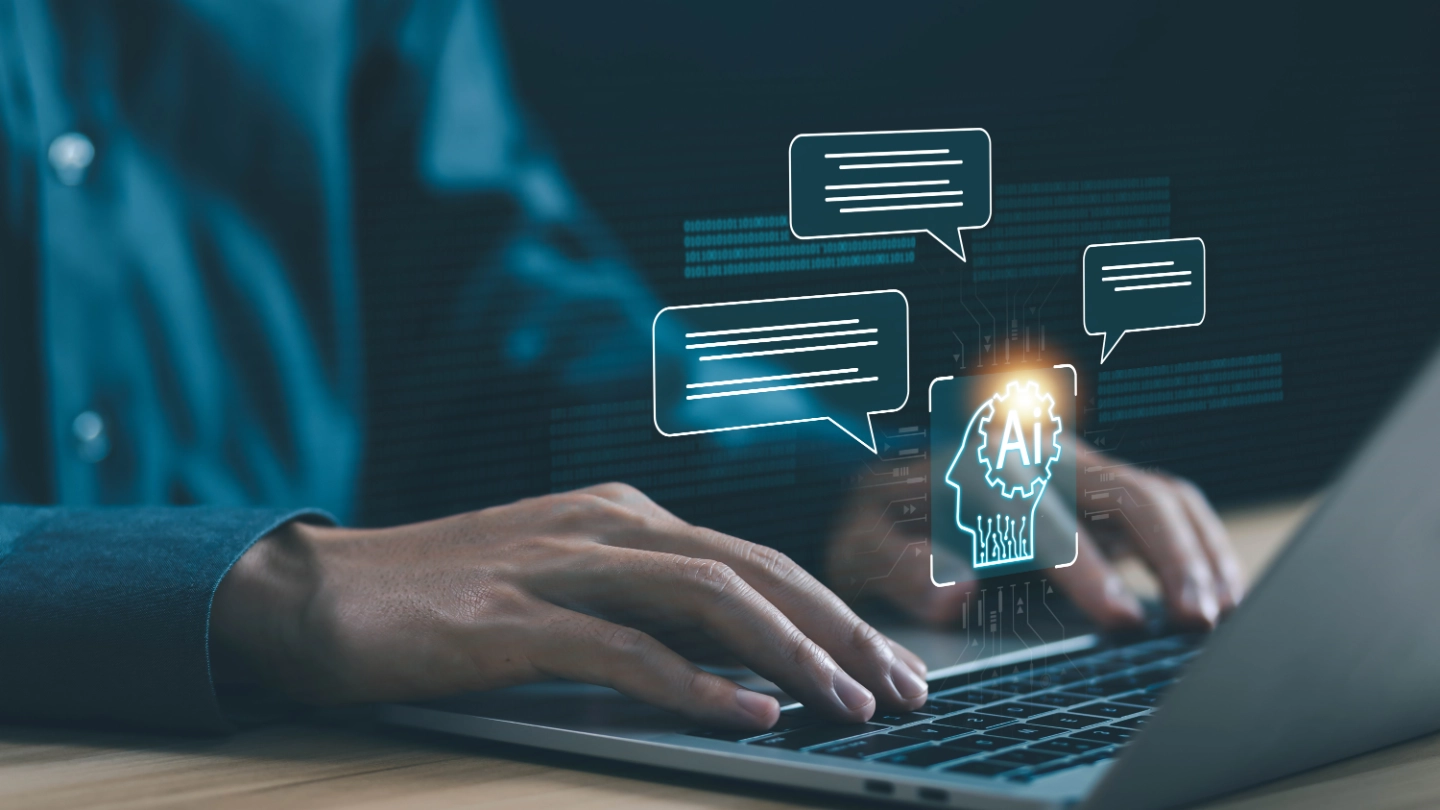
ChatGPT and its derivatives have taken the world by storm. The ability to access the cutting-edge performance of Large Language Models (LLMs) through a simple chat interface has proven to be the killer app for Artificial Intelligence (AI). Billions are being invested by the largest companies around the world to improve upon the performance of our current LLMs to achieve continuously better results. However, it is good to remind ourselves that AI exists in many forms, some of which are more valuable for our uses than others.
At Atradius, we’ve leveraged various AI technologies for nearly a decade to enhance automation and efficiency in our operations. However, since the introduction of ChatGPT, the number of identified use cases has surged dramatically. Fully embracing AI in our industry promises significant innovation and process optimization, but it also requires strategic adjustments. This article delves into how credit insurers can best position themselves to maximize AI’s potential and why this is crucial. Additionally, we explore the opportunities and challenges AI brings to credit insurers, providing insights on how to address these to gain new strategic advantages.
The importance of AI in Credit Insurance
AI's strength lies in its ability to transform vast amounts of high-quality data into variable output for a given purpose – whether that is classification (i.e. decision), generation (e.g., text generation) or something else. Considering the central role that data takes in many credit insurance processes, it is obvious that AI could potentially improve some of the central processes of our value chain.
One basic and central example is the assessment of credit risk, which conventionally involves the meticulous analysis of financial data, credit data, context, and many other diverse sources of information. Traditionally, this process is often complex and time-consuming, requiring the processing of vast amounts of data by humans. AI promises to streamline this process considerably, if the data is available and can be used to train AI models. This allows insurers to assess credit risks on the basis of effectively much more data for each assessment. AI models might also be sensitive to patterns and trends that might be missed by human observers, thus enhancing the precision of risk assessments. In turn, this can lead to much better underwriting performance.
Next to optimised performance, credit insurers can also not only use structured data (e.g., data in the form of tables with titles and descriptions), but also unstructured data, such as news or articles. AI can process this unstructured data much more effectively than years ago. Not only Generative AI (e.g., ChatGPT), but also Optical Character Recognition (OCR) and related technologies can help credit insurers in processing the vast amounts of news, annual reports and other sources that normally would require manual reading and analysis.
Consider that any process for which a good amount of high-quality data is available, could theoretically be optimised to a certain degree by AI. Unlike other industries, where the storage and usage of data is still another hurdle for the adoption of AI, credit insurers particularly with their infrastructure for risk underwriting are almost set up to make use of this new technology to optimise their processes. But there is still work to be done.
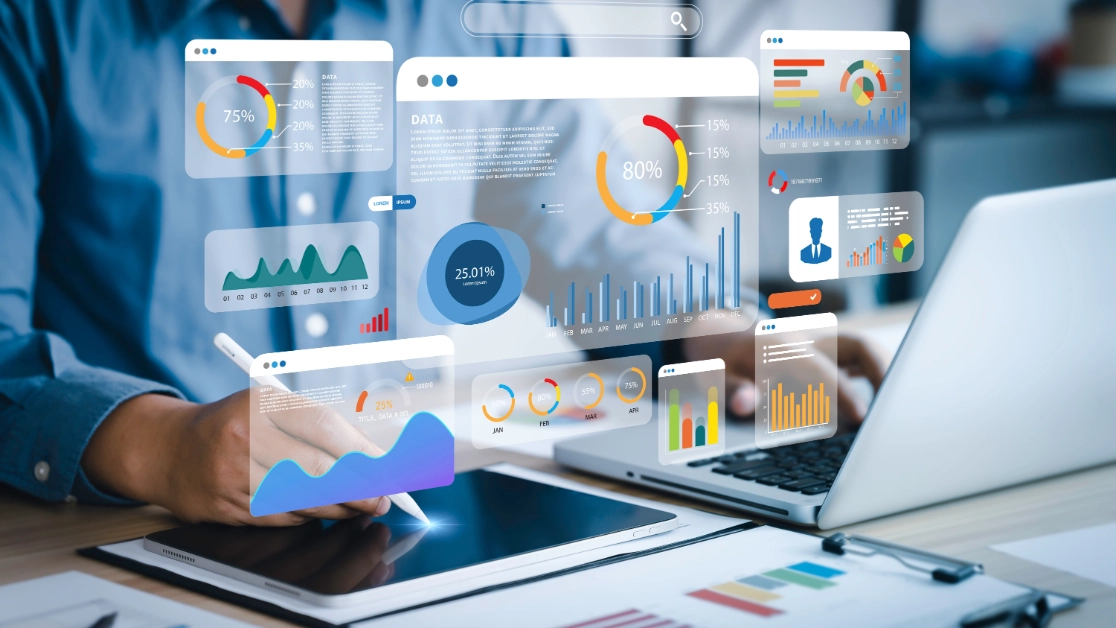
Preparing for AI: A strategic imperative
Adopting AI involves more than just investing in new technologies. It requires a shift throughout strategy, operations, and culture. Credit insurers must recognise that AI is not simply a tool but a fundamental shift in the way business is conducted. The strategic imperatives fall into three main categories: data strategy, investment in technology, and upskilling the workforce.
The importance of a data strategy to train effective AI models
At the start of any AI solution is data. AI algorithms need to be trained on extensive datasets in order to generate accurate insights, predictions, and decisions. However, raw data on its own is not enough; it needs to be organised, cleaned, and made accessible in a way that AI models can be trained effectively. This is where a data strategy becomes indispensable. A well-defined data strategy will ensure that the right types of data are collected, stored securely, and managed consistently.
For example, AI models might use financial data, economic indicators, historical default rates, and customer profiles to be trained. Ensuring this data is standardised and correctly labelled allows the AI to draw relevant insights and deliver high-precision credit risk assessments. Insurers that adopt an ad-hoc or piecemeal approach to data management will struggle to gain reliable insights from their AI investments. A data strategy in practice will also often imply investments in underlying technical infrastructure, whether that is in cloud computing, data lakes or data analytics platforms.
Investment in technology
To prepare for AI implementation, it’s crucial to establish a robust technological infrastructure. Training AI models demands significant computational power, which can be provided by specialized computing clusters or cloud computing services. Insurers should leverage existing advancements by tech companies to lower the entry barriers for AI adoption. Beyond acquiring new systems, insurers must create an integrated technological ecosystem. This ecosystem should allow AI to seamlessly access and analyse data from current platforms and databases, enabling it to generate insights that enhance decision-making processes. Moreover, insurers must ensure that their data governance policies are robust. AI thrives on data, but the quality and accessibility of that data are crucial. Insurers will need to invest in cleaning, structuring, and managing their data in a way that maximises its utility for AI applications. This also includes addressing issues around data security, privacy, and regulatory compliance, as these will be critical in maintaining trust with clients and meeting legal requirements.
Upskilling the Workforce
While AI can automate many tasks, it does not replace the need for human expertise. On the contrary, AI's introduction will elevate the need for a workforce skilled not only in data analysis and AI systems management, but also the adequate use of AI-powered systems. Credit Insurers must invest in upskilling their employees to work alongside AI, such as underwriters interpreting AI decisions in the right context and conscious of its limitations. Given the niche nature of our industry, this will often involve training existing staff rather than recruiting new talent with specialised skills.
Additionally, a cultural shift will be needed within organisations. This mindset shift is crucial, as AI can often be associated with job loss and other criticisms that are not always warranted. This kind of change resistance to technological change can impede otherwise well-performing AI initiatives. Credit Insurers that foster a culture of innovation and continuous learning will be better positioned to take full advantage of AI's potential.
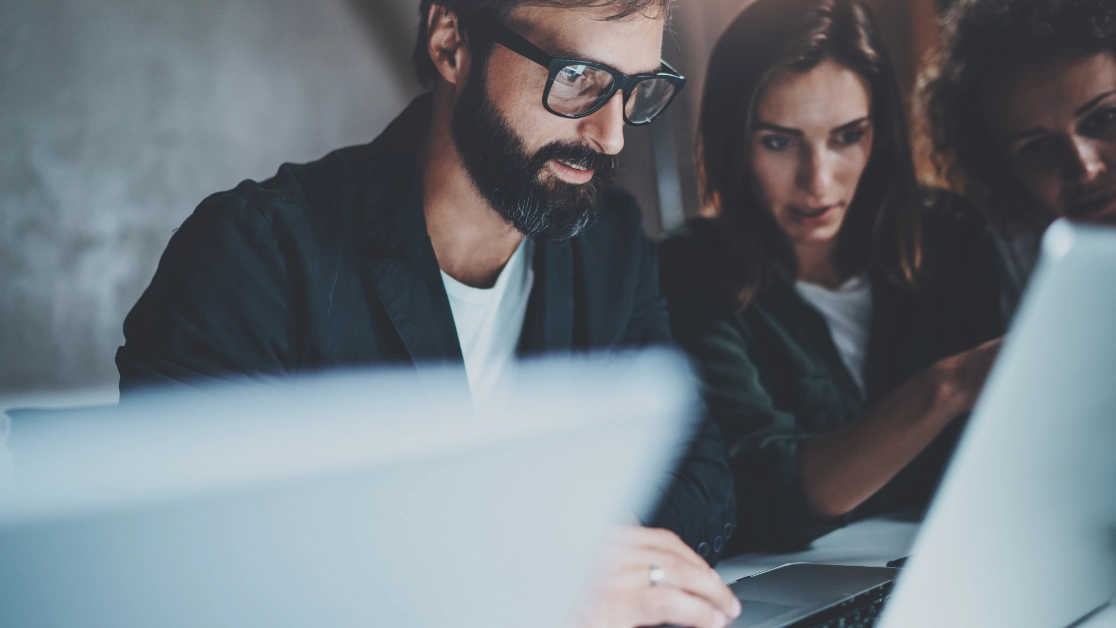
Opportunities of AI
AI presents a wealth of opportunities for credit insurers willing to embrace it. One of the most immediate benefits is the ability to improve risk assessments through predictive analytics. AI can analyse vast datasets, including economic indicators, and potentially even social media sentiment to predict which companies are most likely to default on their payments.
Another opportunity lies in automating claims processing. Traditionally, processing a claim can be time-consuming and resource-intensive, often requiring significant documentation and manual verification. AI can automate much of this process, verifying claims data against third-party sources and even detecting fraudulent claims through pattern recognition algorithms. This can reduce costs for insurers, speed up payouts for customers, and improve overall operational efficiency.
AI also offers new ways of engaging with customers. For instance, AI-driven chatbots can provide policyholders with instant answers to their queries and assist in the claims process. By automating routine customer service tasks, insurers can free up employees to focus on more complex and high-value interactions.
Challenges for AI
While AI brings significant opportunities, it also presents notable challenges that insurers will need to address. One challenge is adhering to regulatory requirements. AI systems handling personal or financial data must comply with data protection laws and upcoming AI regulations like the EU’s AI Act. This act mandates a risk assessment for AI systems, risk management tailored to each use case, transparency about AI usage, and stringent data governance practices. Insurers must ensure their AI systems fully comply with these regulations and have strong data governance frameworks.
The threat of cyberattacks also remains. As with any digitalisation, credit insurers must keep investing in cybersecurity measures to protect their systems from malicious actors. This will require ongoing vigilance, as cybercriminals are continually evolving their tactics, some also enhanced by AI.
We must also be weary of the AI delusion. As some rush to implement GenAI into business processes, often driven by fear of missing out on opportunities, we forget that it is often not best suited to solve every conceivable issue. Other tested technologies still remain relevant, and in many cases a sound combination of different tools is significantly more performant than any single technology used in isolation. Finally, there is the challenge of customer trust and understanding. As AI takes on a greater role in decision-making, some customers may feel uneasy about the perceived lack of human oversight. Credit Insurers will need to maintain transparency about how their AI systems work and offer customers clear explanations of how decisions are made. Building trust will be essential to ensure that AI-driven processes are accepted by the market.
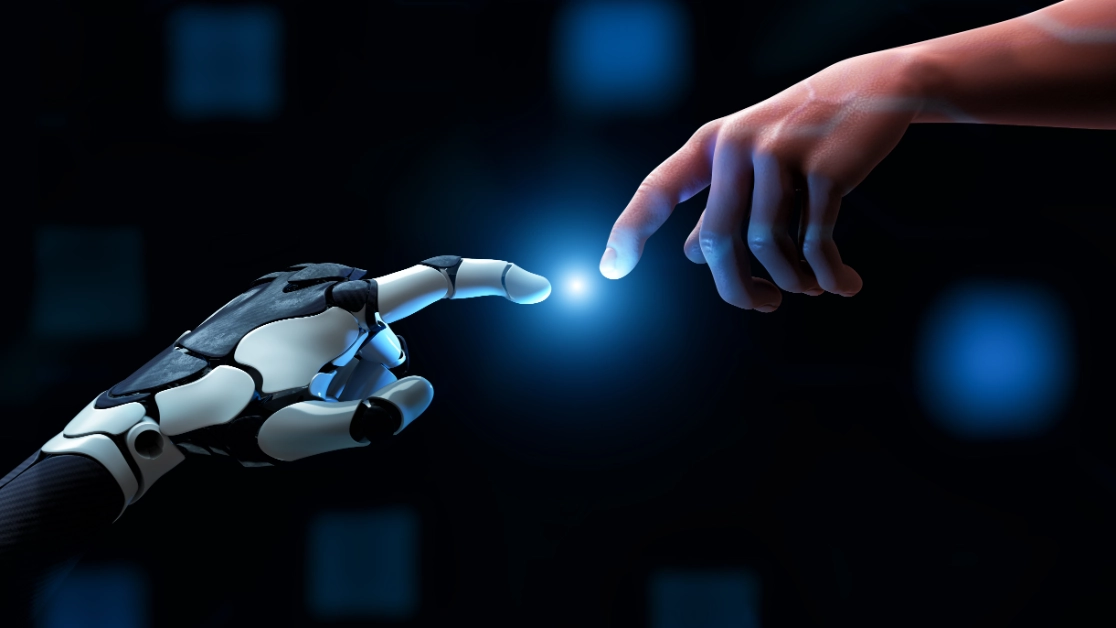
Conclusion
The future of credit insurance is undoubtedly intertwined with AI. Given all the prerequisites and preparations for AI usage throughout the credit insurance organisation, it is not a completely straightforward process.
We have not described the full potential for AI in credit insurance and we are unlikely to be able to already predict the full impact that AI will have on the industry. However, it is clear that it bears great potential that is ready to be leveraged by credit insurers around the world.
Source: ICISA Insider